At Ivy, we have been building newer technology for consumer goods companies for the past 20 years. Our R&D teams have created many solutions to help consumer goods companies scale and keep pace with the increasing competition. Thanks to advances in artificial intelligence, machine learning, and deep learning, you can now analyze millions of stores using a data model to understand behaviors and trends to make recommendations. Read on to find out how recommender systems help you learn more about your customers and boost sales.
This blog is also a walk-through down the memory lane of Ranjith T, Head of Product Development, and explains what went behind developing our native recommendation engine- Ivy Recommender.
Prescriptive Over Predictive
A typical sales recommendation engine analyzes the spectrum of data to offer prescriptive advice to sales reps on the actions to take. These are a step ahead of predictive because predictive can tell what will happen, but prescriptive can change what will happen if you act in a particular way.
We all encounter recommendation engines in our day-to-day lives like movie suggestions on Netflix, product recommendations by Amazon, and many others. That is all B2C! For consumer goods companies, where sales reps visit stores to push product sales, we have applied the same analytical wisdom to the needs of consumer goods companies. Our solution can help them derive more value from each channel and augment sales rep decisions for better performance.
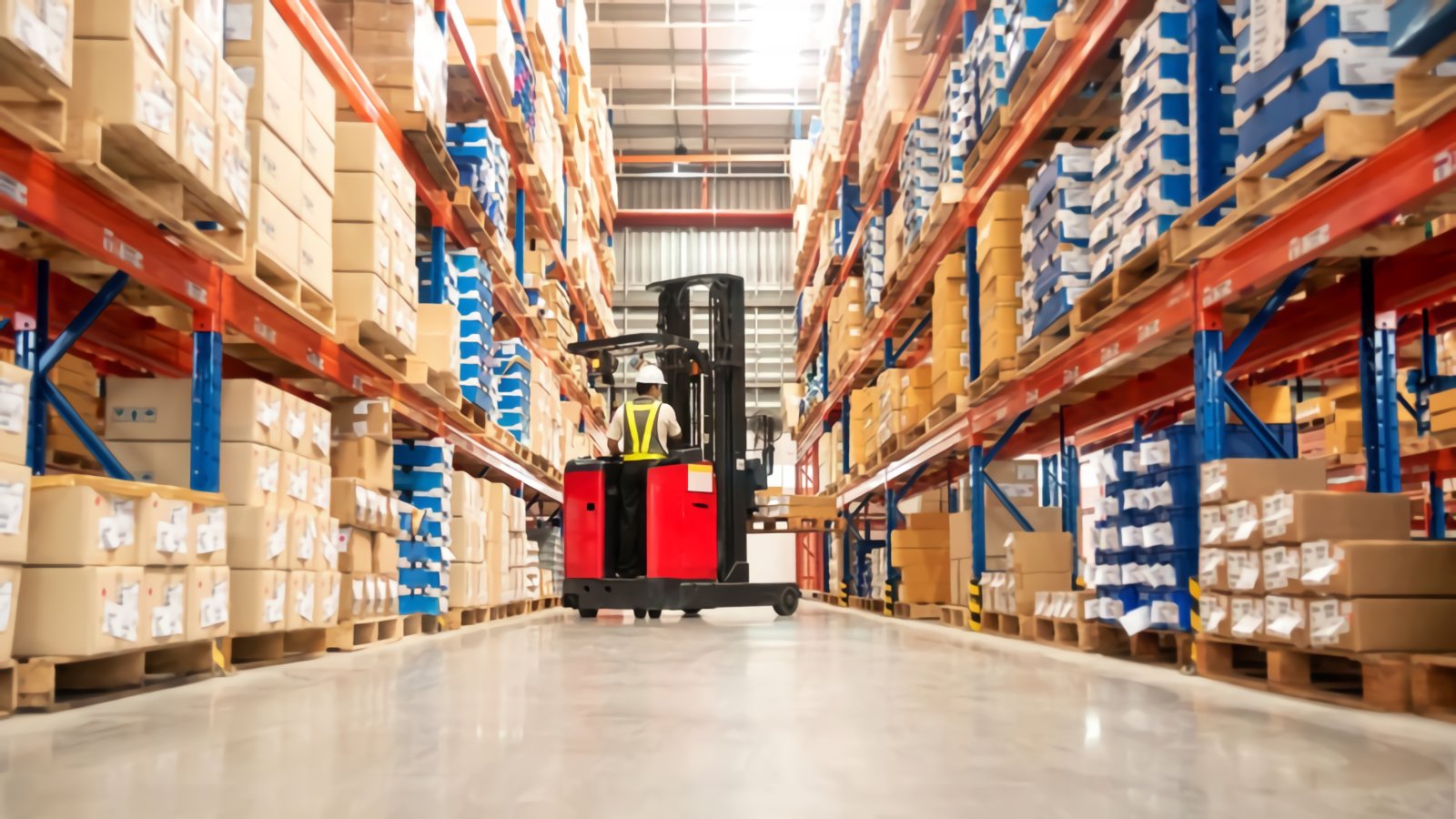
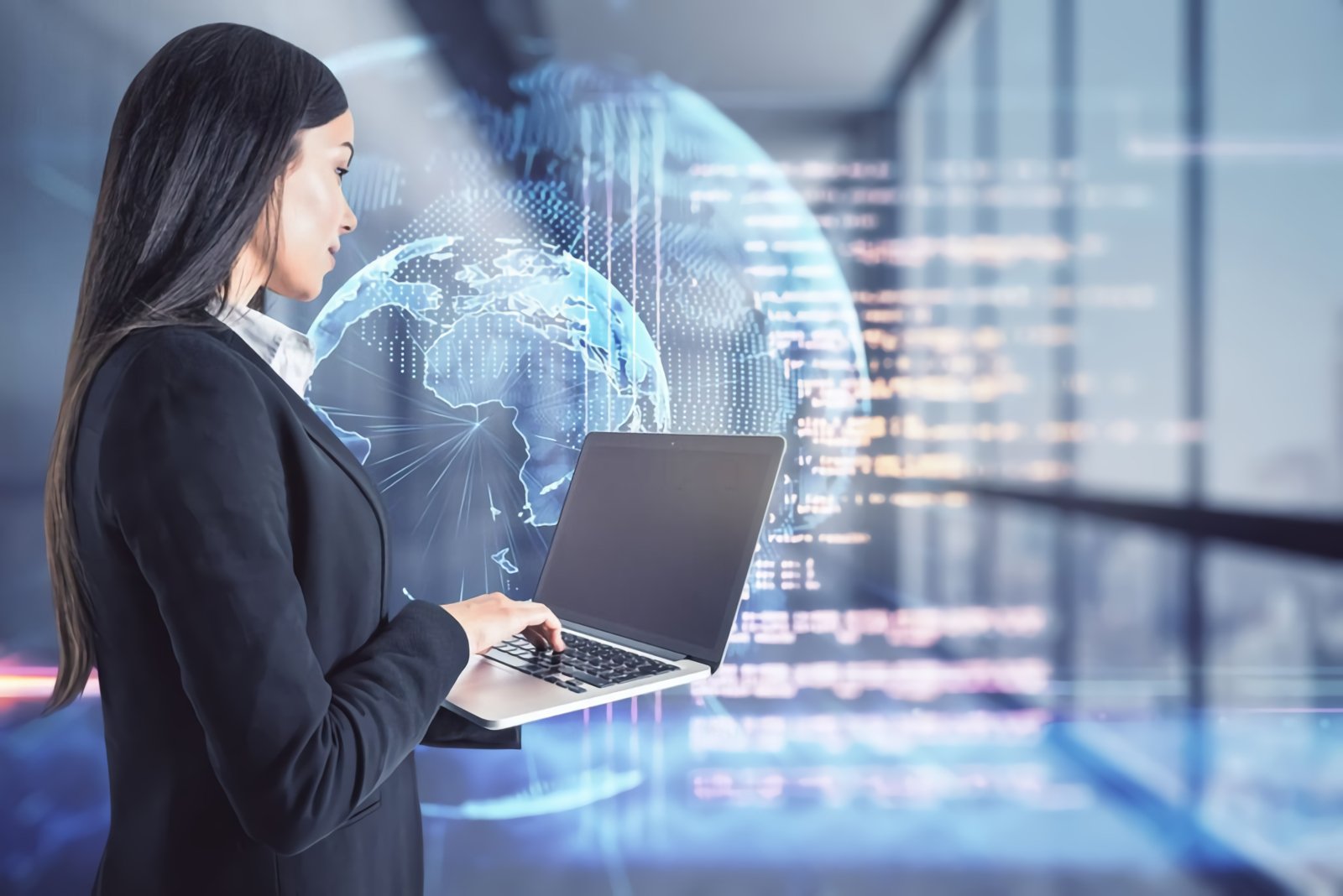
Fitting into Distribution Operations
You may wonder that this all sounds great in theory, but how does it fit into practical applications. A consumer goods company with an excellent sales team, where sales reps have great relationships with every store, would also benefit from a recommendation engine.
Imagine a scenario where your sales reps share recommendations with store managers and owners, and the store is impressed with the amount of information your team has at their fingertips. What would be even more exciting is when the store benefits from these recommendations. You get better customer experience, growth, perfect in-store execution, fewer stockouts, and large orders. It also implies more revenue, an increase in same-store sales, and an improvement in profit margin.
Recommendations for the WinÂ
A Harvard Business Review study suggests that recommender systems are an important algorithmic distinction between born-digital organizations against legacy firms. It also mentions that when AI & ML generates personalized recommendations by analyzing customer data, it can increase sales for retailers by 1% to 2%, and our native recommender solution, Ivy Recommender, has the potential to increase sales for retailers by 5%.
We built our Recommendation Engine to help organizations derive better value from their data to help them scale their business and improve sales. One of our biggest wins is that our solution only needs a meager six months of past data to generate recommendations with high precision and recall values. However, we prefer that our customers use data of at least 13 months to ensure that the seasonality aspect is covered.
Close to Perfection
Recycling and reusing multitrack records has helped us create repeatable patterns that save time for our recommendation algorithm to produce results. We now offer two significant recommendations with focused improvements: Fit Sell and Up-Sell. We also have 14 other use cases in the pipeline that would essentially mitigate problems from recruitment to performance of field agents.
The more you use the recommender, the more it learns and improves. Recommenders use massive computational power to process millions of gigabytes of data. It also runs parallel threads to process several hundred parameters, segment indexes, permutations, and combinations to identify patterns amongst this data.
The Future of Recommenders
The journey towards innovation is underway. We shadow sales reps on their trips to the stores to understand how our recommender makes a difference in their job. That helps us get insights into how to enhance what they do and design accordingly.
As we learn more about our engine, we are enhancing existing features while developing new revenue growth management packages that come with an assurance of increased profitability. We also hope to build a system that can train and enhance the productivity of our field force agents.
The other part on which the future of recommenders depends is usability. If a consumer goods company implements the recommender, but the operations team doesn’t understand its value, it won’t serve the purpose. Also, if the recommendations do not load quickly, the sales rep may lose faith in it and will do things the old way. Hence adoption and solution performance go hand-in-hand. If a sales rep feels that Ivy Recommender helps me finish my day sooner and also helps me meet my targets, it would be a win for us and a compelling statement for consumer goods companies to adopt it.
In closing remarks, Ranjith says, “At Ivy, we strive to help consumer goods companies build a deeper, robust, and valuable relationship with its channel partners- a relationship that translates to mutual growth and win-win for both.”